Introduction
This FAQ sets out BSR’s perspective on generative AI and its implications for ethics and human rights. It offers guidance to both the developers of generative AI (e.g., technology companies) and the deployers of generative AI (e.g., both technology and non-technology companies) for ensuring a responsible, safe, and rights-respecting approach to developing and deploying generative AI systems.
Definitions
What is Generative AI?
Generative AI is a type of machine learning, a field of computer science focused on building systems that learn patterns and structures in existing data. Generative AI models are trained on massive amounts of data, which enable models to recognize patterns and structures in the data or understand meaning in words or phrases. Generative models use this knowledge to generate new content in the form of text, image, audio, video, code, or other forms of media in response to user prompts. Commonly known generative AI technologies include chatbots, such as Chat GPT and Google Bard, and image-generating technologies, such as Adobe Firefly and Midjourney.
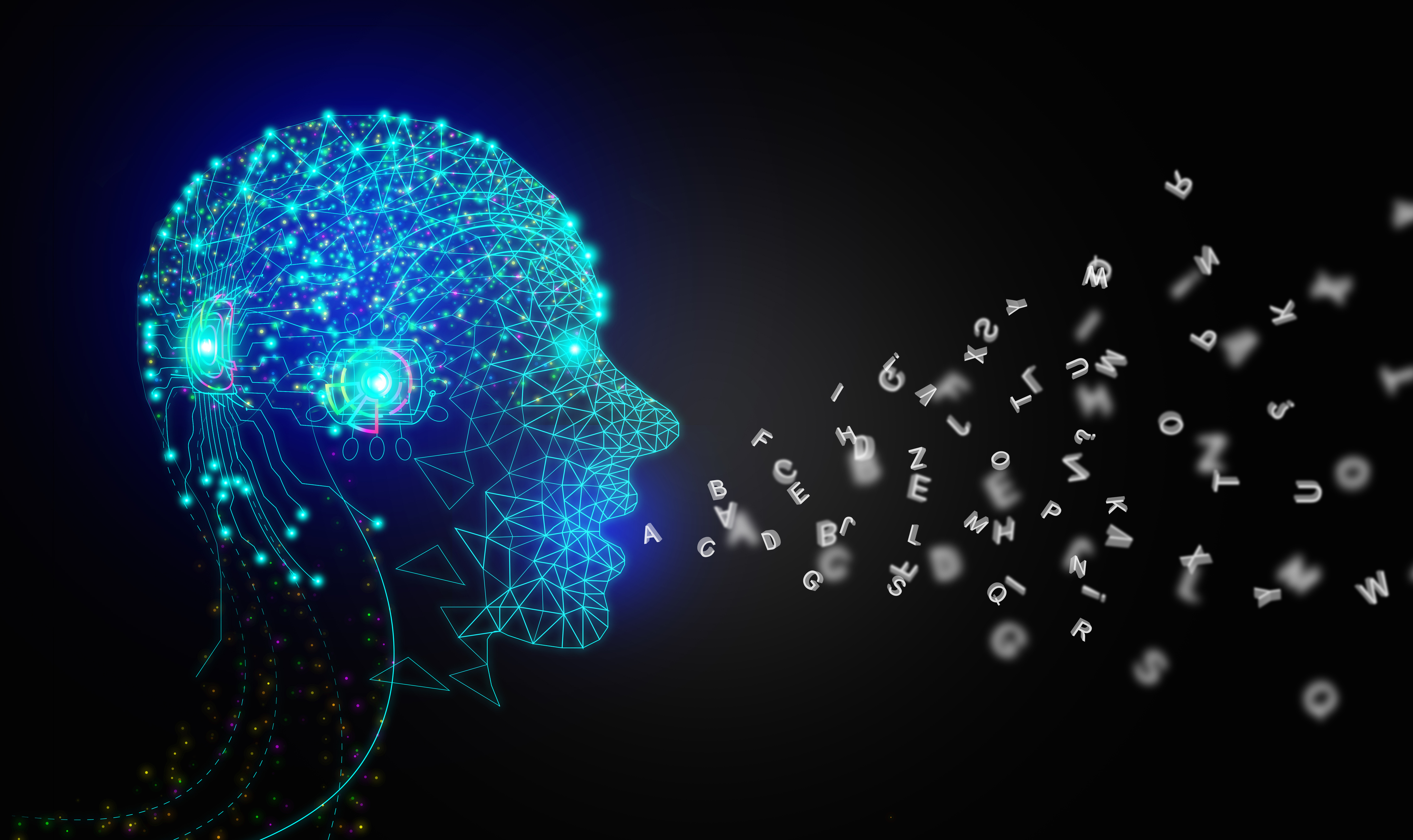
Some generative AI models rely on a training method called reinforcement learning through human feedback (RLHF). This method relies on human labelers to assess generative AI outputs for their quality. In their evaluation of model outputs, labelers may consider accuracy, toxicity (e.g., misinformation, hate speech, etc.), and/or adherence to specified principles (e.g., non-discrimination, political neutrality, reducing bias in outputs, etc.). This feedback is incorporated into the model to improve model performance and to prevent models from generating harmful outputs.
Risks of Generative AI
Where do risks in relation to generative AI exist in company value chains?
Risks exist pertaining to both (1) the design, development, training, and optimization of generative AI models, and (2) the deployment of generative AI models.
Are generative AI risks relevant for the long or short term?
Risks relating to generative AI exist in both the near-term and the long-term. Companies should focus on current and near-term concerns, while maintaining sight of potential long-term impacts associated with the adoption of generative AI at scale; addressing real adverse impacts in the near-term will help companies, governments, and society prepare for long-term impacts.
What are the main risks pertaining to design, development, training, and optimization of models?
Some of the main categories of near-term risk are as follows.
-
Coded bias: Generative AI models may be trained on datasets which reflect societal biases pertaining to race, gender, or other protected characteristics. Generative AI models and future technologies trained on generative AI foundation models may reproduce the biases encoded in the training data.
-
Linguistic limitations: Generative AI models are currently not available in all languages. Furthermore, there may be discrepancies in model performance across languages and dialects in which models are currently available. Linguistic limitations may lead to increased digital divides for speakers of low-resource languages or linguistic minorities.
-
Low interpretability: Generative AI models may have low levels of interpretability, meaning it is challenging to determine with full transparency how models make the decisions they make.1 While techniques are being developed to increase transparency of AI decision-making, low interpretability creates current and near-term risks.2 Low interpretability may pose challenges for diagnosing and correcting issues in the model. For example, if a model were consistently producing inaccurate or discriminatory outputs, it may be hard to understand why it is doing this, making it challenging to correct the issue. This increases the potential for harm when deploying models in high-risk contexts, such as autonomous driving or criminal justice.
-
Implementing changes to models may be time-consuming: For models which rely on RLHF, the process of improving model outputs or implementing changes at scale may be time-consuming. While techniques are being developed to automate this process, the reliance on human labelers creates current and near-term risks.3 For example, the issue of a model producing harmful outputs may not be quickly addressable, and those harmful outputs may be widely disseminated, increasing their potential to create adverse impacts.
1 Interpretable Deep Learning: Interpretation, Interpretability, Trustworthiness, and Beyond
2 Interpretability in Deep Learning
3 Constitutional AI: Harmlessness from AI Feedback
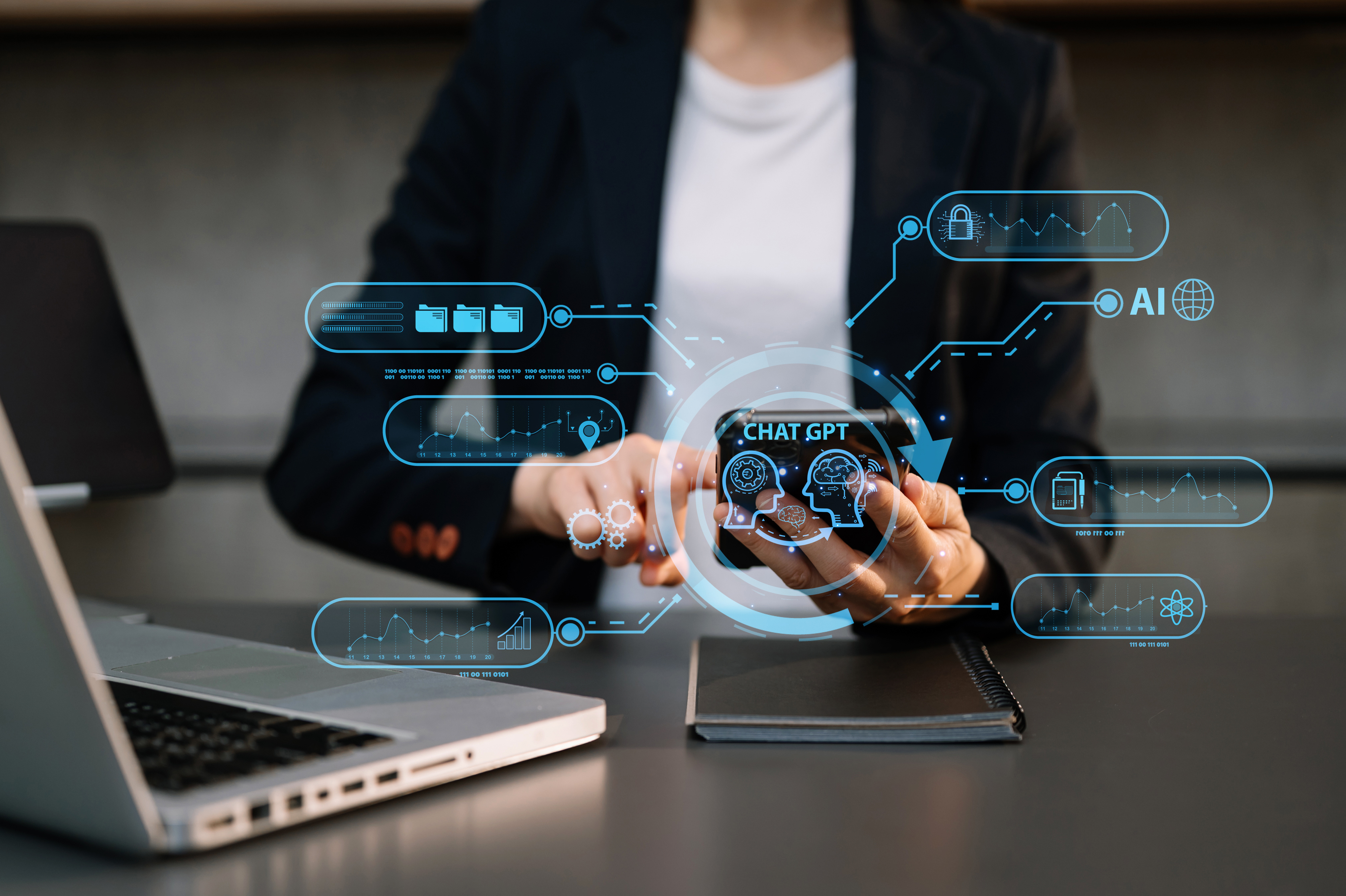
What are the main risks pertaining to deployment of models?
Some of the main categories of near-term risk are as follows.
-
Harms associated with jailbreaking: Jailbreaking, in this context, is when a user breaks the safeguards of a generative AI model, enabling it to operate without restrictions. This allows the model to produce outputs that may otherwise violate its programming. As generative AI models are deployed in increasingly high-risk settings, jailbreaking models may come with increased consequences or potential to cause harm. For example, jailbreaking models integrated into military technology may have severe impacts.
-
Harmful outputs: Even with safeguards in place, generative AI models may produce outputs which are inaccurate, biased, nonrepresentative, plagiarized, unsourced, not relevant to the prompt, or otherwise harmful. The severity of the consequences associated with harmful outputs depends on the context in which the model is deployed. For example, for models deployed in the context of medical diagnosis, inaccurate outputs may be associated with greater adverse impacts.
-
Harmful end uses and model manipulation: Generative AI models may be deployed for harmful end uses, and models may be manipulated to behave in harmful ways or to conduct harm at scale. For example, generative AI may be used to design and execute sophisticated and convincing scams or to spread disinformation rapidly at scale.
-
Privacy: Generative AI has implications on privacy including concerns pertaining to the collection and storage of data, how data may be resurfaced by models, how models may make accurate (or inaccurate) inferences about a user, or how outputs may re-identify users from anonymized data.
Do open or closed source generative AI models present greater risk?
There is active debate about the risks and benefits of “open versus closed source” generative AI models. Open-source AI models are made freely available to the public for distribution, modification, and use; closed source models are not widely nor freely available to the public, with access, distribution, and use typically controlled by the organization or company that developed them.
Open-source models may enable contributions, research, and knowledge-sharing from diverse groups of stakeholders, but may also increase the potential for misuse, abuse, or the amplification of bias or discrimination.
Closed-source models may provide greater control and protection against model misuse or abuse, but this may come at the expense of limiting transparency and the ability of researchers, developers, and the public to understand and assess the underlying data, algorithms, and decision-making processes of the model.
What are the main long-term risks and large-scale impacts?
Some of the main categories of longer-term risks are as follows, though many of these are also happening today. This FAQ does not cover longer-term existential questions (e.g., is AI a threat to the future of humanity) since they are largely irrelevant for business decision making today and divert attention away from more pressing risks.
-
Worker displacement or the devaluing of labor: The adoption of generative AI across industries may be associated with the displacement of workers, increased unemployment, the devaluing of certain types of labor, and the exacerbation of wealth disparity. The labor market will need to evolve in response to the deployment of generative AI models across industries, and displaced workers may have to develop new skills or transition into new opportunities or roles.
-
Proliferation of misinformation: The deployment of generative AI models may contribute to the proliferation of mis- /disinformation, particularly due to the technology’s capability to produce high volumes of “credible” content quickly. This may be salient during elections and key periods of civic engagement or unrest. The creation and spread of mis- /disinformation is likely to evolve, necessitating a mix of effective technical mitigations (e.g., content labeling, cryptographic provenance) and social mitigations (e.g., media literacy).
-
Widespread distrust of media and the truth: The proliferation of highly realistic synthetic media (audio, image, video, etc.) may affect public perception of media. In an age when “seeing is no longer believing,” this may impact the ability of communities and societies to agree on what is truthful and real.
-
Negative health outcomes: Engaging with generative AI models, or harmful content produced by generative AI models, may have impacts on mental health outcomes of users including increased instances of mental illness and self-harm. Generative AI models may amplify problems of targeted harassment, bullying, or false impersonation online, and some individuals or groups may be subject to harassment or bullying over others.
Is this a complete list of risks?
No. Generative AI may pose risks to several other areas including: indigenous people’s cultural and/or property rights (models may plagiarize original works by indigenous artists or writers); rule of law (mis-use of generative AI in the criminal legal system); peace and security (the proliferation of synthetic mis- /disinformation depicting national security threats may result in real-world harms); digital divide (inequitable access to generative AI and the benefits it engenders may exacerbate the digital divide across regions and languages); and others.
Opportunities of Generative AI
What are the main opportunities associated with generative AI?
There are potential opportunities for nearly all industries and society more broadly. These opportunities do not offset the risks (e.g., all risks should still be addressed even if benefits outweigh them) but do provide a rationale for the pursuit of generative AI.
-
Creation of meaningful work opportunities: Generative AI models can be deployed to augment human activities and contribute to the development of new jobs or opportunities. If generative AI technologies are used to complement human labor or create new tasks or opportunities, then demand for labor may increase and / or higher quality jobs may be created.
-
Increases in productivity and innovation: Generative AI has been hailed for its ability to drive transformation and productivity and may be the basis of widespread technological innovations. Increases in innovation and productivity may be associated with broadly shared prosperity and increased standards of living.
-
Detection of discriminatory or harmful content: The capabilities of generative AI models may make them powerful automatic detectors of harmful or discriminatory content online, thereby improving online content moderation and reducing reliance on human content moderators who often experience psychological impacts as the result of viewing harmful content.4
-
Access to information and education: Generative AI may improve the information ecosystem by providing access to high quality information in a speedy manner and in an accessible format. This may support the educational experience, particularly if models are adaptable to the capacities or learning styles of different users such as children or neurodivergent users.
-
Accessibility solutions: Advances in technology, such as mobility devices and disability assistive products and services, enable increased quality of life for persons with disabilities. Generative AI may also provide greater accessibility options to persons with disabilities through use cases that address their specific needs.
-
Access to health: Generative AI may accelerate the development of health interventions, including drug discovery and access to health information that supports basic needs.
Company action
What frameworks can be used to assess generative AI?
Companies developing and deploying generative AI should do so in a manner consistent with their responsibility to respect human rights set out in the UN Guiding Principles on Business and Human Rights (UNGPs).
The UNGPs offer an internationally recognized framework (e.g., human rights assessment) and a well-established taxonomy (e.g., international human rights instruments) for assessing risks to people. Furthermore, the UNGPs provide an established methodology for evaluating severity of harm, prioritizing mitigation of harm based on severity, and navigating situations when rights are in tension. This enables companies to determine appropriate action for mitigating potential harms associated with their business operations.
Human rights frameworks may be used in combination with existing AI ethics guidelines, either industry-wide guidelines or individual company guidelines, to inform companies’ approaches to ethical generative AI development and deployment. Existing AI ethics frameworks include:
Does responsibility for addressing risks reside primarily with the developers or deployers of generative AI?
Companies across industries are deploying generative AI solutions, and the responsibility of mitigating risks resides with both developers and deployers of generative AI systems. Some risks may be best addressed by developers (e.g., risks associated with model creation) while other risks may be best addressed by deployers (e.g., risks associated with the application of the model in real life). Many risks will benefit from collaboration between developers and deployers (e.g., refining the model and improving guardrails in response to real world insights). BSR offers the following considerations.
Developers (e.g., technology companies)
-
Industry-wide collaboration: Companies developing generative AI models can engage in industry-wide collaborative efforts, such as Frontier Model Forum, to align on ethical and rights-respecting standards for developing, deploying, and selling generative AI models.
-
Generative AI-specific principles: Companies may develop new principles to guide ethical approaches to developing generative AI models or may apply existing AI principles and tailor or expand them to account for the unique challenges and risks posed by generative AI systems.
-
Ongoing due diligence: Conduct ongoing due diligence on evolving generative AI models and integrations with increased sophistication and capabilities. This includes human rights due diligence and model safety and capability evaluations, such as red teaming and adversarial testing.5
-
Research: Fund research into real and potential societal risks associated with the adoption of generative AI and continued explorations of responsible release. Research efforts should seek to design mitigations for the risks identified, including evolving methods for increasing model interpretability and accountability, reducing bias and discrimination in models, and protecting privacy.
-
Transparency and reporting: Publicly report on model or system capabilities, limitations, real and potential societal risks, and impacts on fairness and bias. Companies should also offer guidance on domains of appropriate and inappropriate use.
-
Customer vetting: Before providing off-the-shelf or customized generative AI solutions, vet potential customers to ensure their intended use case or possible end uses of the technology will not lead to harm.
Deployers (e.g., both technology and non-technology companies)
-
AI ethics principles: Companies seeking to implement generative AI solutions should ensure that they have robust AI ethics principles if they do not already. AI ethics principles should account for the specific risks posed by generative AI, as well as AI more generally. Principles should set standardized, right-respecting approaches for the adoption of AI systems across all areas of the business.
-
Human rights due diligence: Prior to implementing customer-facing or internal generative AI solutions, conduct human rights due diligence to identify the potential harms that may result from the deployment of the technology.
-
Mitigation: Implement guardrails to address risks surfaced in the human rights due diligence process. For customer-facing or employee tools built on generative AI, ensure there is a reporting channel for customers and employees to report issues with model performance.
-
Consent: Obtain user or customer consent before training models on their data or content. Give users or customers the option to opt out of having their data or content used to train models (for example, allowing artists to opt out of having their artwork used to train image generating models).
-
Continuous monitoring and evaluation: Regularly assess training datasets and the performance of generative AI systems to evaluate them for fairness and bias. Integrate feedback from reporting channels on an ongoing basis.
Let’s talk about how BSR can help you to transform your business and achieve your sustainability goals.